When to implement an ML monitoring solution
Monitoring is crucial to ensuring that ML models deployed in production are serving their intended purpose and operating as expected, but how soon is too soon to implement a monitoring solution? Ultimately it depends on the extent to which the models are integrated into business processes, and this blog post will walk through the considerations that should be made before deciding to implement an ML monitoring solution.
What is ML Monitoring?
ML monitoring is about gaining proactive visibility and intelligence into ML applications, models and data pipelines. The overarching objective of such monitoring is to detect issues in those applications, models or pipelines before downstream business KPIs are negatively affected. In another post, we have delved into how to evaluate complete monitoring solutions and separate them from the “pretenders”. This post focuses on the timing of implementing an ML monitoring solution. Doing so too soon can unnecessarily hamstring your R&D efforts, but waiting too long can lead to hugely detrimental flaws in your AI system to propagate to your business processes.
Your Business Is Relying on ML Models
If your business is already relying on ML models to further a crucial business process such as detecting fraud, managing dynamic pricing, or predicting customer engagement, then the best time to have implemented ML monitoring was yesterday. Every model that is living inside the production environment and interacting with customers or business processes should have monitoring layered on top of it. Without monitoring, it is impossible to tell how the model is affecting downstream business KPIs. It could be that a poorly calibrated model is actually leading to non-negligible customer attrition or causing a loss in revenue to the business. Less fundamental problems might also exist, such as a model that frequently experiences downtime or experiences a high load, leading to large latency times for customers accessing the web service which it is a part of. Regardless of the exact nature of the potential failure, insight into any potential problems that may arise is critical to isolating them before they propagate into major losses for your business.
Even if your rules-based business process is not yet fully automated, and you are only experimenting with integrating ML into parts of it, there can be substantial value in implementing ML monitoring in tandem with this experimentation. Having visibility into how the ML model is inducing change into, or how it is interacting with, the rest of the business process will allow you to understand whether the model is actually serving its intended purpose and increasing the feasibility of the business process.
Anecdotally, this practice is followed by major tech companies. For example, when allocating cloud compute resources for running services, Google gives monitoring jobs a higher priority than the actual processes they’re monitoring! This ensures that a model is never running in production without proper monitoring infrastructure in place.
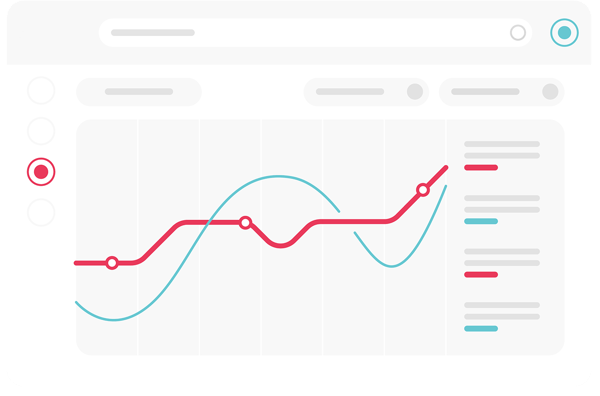
Your Data Management is Messy
Maybe you don’t have a fully functional data lake yet, or maybe you are still working on establishing the best ETL procedures for your exact types of data. If this describes your organization, you might be wondering whether it’s best to address those weaknesses first, prior to focusing on implementing an ML monitoring solution. The answer is that it depends on your current level of reliance on ML models. If your business has already integrated machine learning into its business processes, then it’s more important to focus on getting a monitoring solution up and running as this will have the most immediate and significant impact. After all, your business is leaning on these models to deliver actionable insights that directly affect your customers, your reputation, and your bottom line. In this case, it’s best to introduce monitoring as your highest priority in order to ensure that you are getting the most out of your models and to determine that there are no hidden issues lurking under the surface.
That said, if your business is not yet reliant on ML modeling because you are waiting on establishing the data ingestion processes necessary to train and serve those models, then it makes sense to focus your efforts towards getting your data management processes streamlined and working.
You Are in the Research or Experimentation Stage
If you’re still just experimenting with ML models in a non-production environment, i.e. your customers and internal business processes are not relying on them, then it might be too early to be considering a monitoring solution. You’ll want to ensure that you’ve identified good use cases for ML modeling, selected the best training datasets, and determined the means by which you will offload those models into the production environment. Only after you’ve addressed these initial roadblocks and settled on a roadmap for incorporating modeling into your business processes should you begin implementing an ML monitoring solution.
Ready to implement ML monitoring?
If you’ve decided that you’re ready to implement ML monitoring, then you’ll want to take these initial steps to get off to the best possible start. First, establish ML performance metrics that will guide your monitoring. Some of the best metrics include feedback from humans-in-the-loop or directly measurable business KPIs. Then, when selecting a monitoring solution, you’ll want to make sure it delivers on these three key features: complete process visibility, proactive, intelligent ML monitoring insights, and total configurability. With the proper monitoring tools in hand, your business can be assured of full visibility into its ML modeling pipeline. Have any questions? Reach out to us to discuss your ML monitoring needs!