Data drift, concept drift, and how to monitor for them
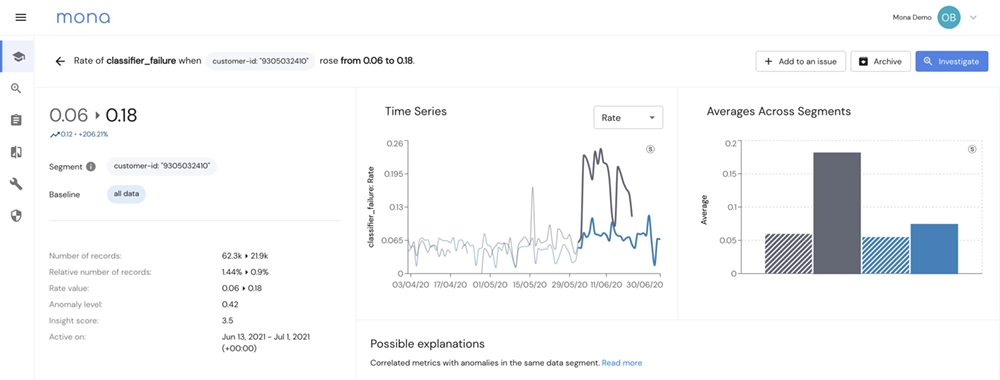
Data and concept drift are frequently mentioned in the context of machine learning model monitoring, but what exactly are they and how are they detected? Furthermore, given the common misconceptions surrounding them, are data and concept drift things to be avoided at all costs or natural and acceptable consequences of training models in production? Read on to find out. In this article we will provide a granular breakdown of model drift, along with methods for detecting them and best practices for dealing with them when you do.