Is your LLM application ready for the public?
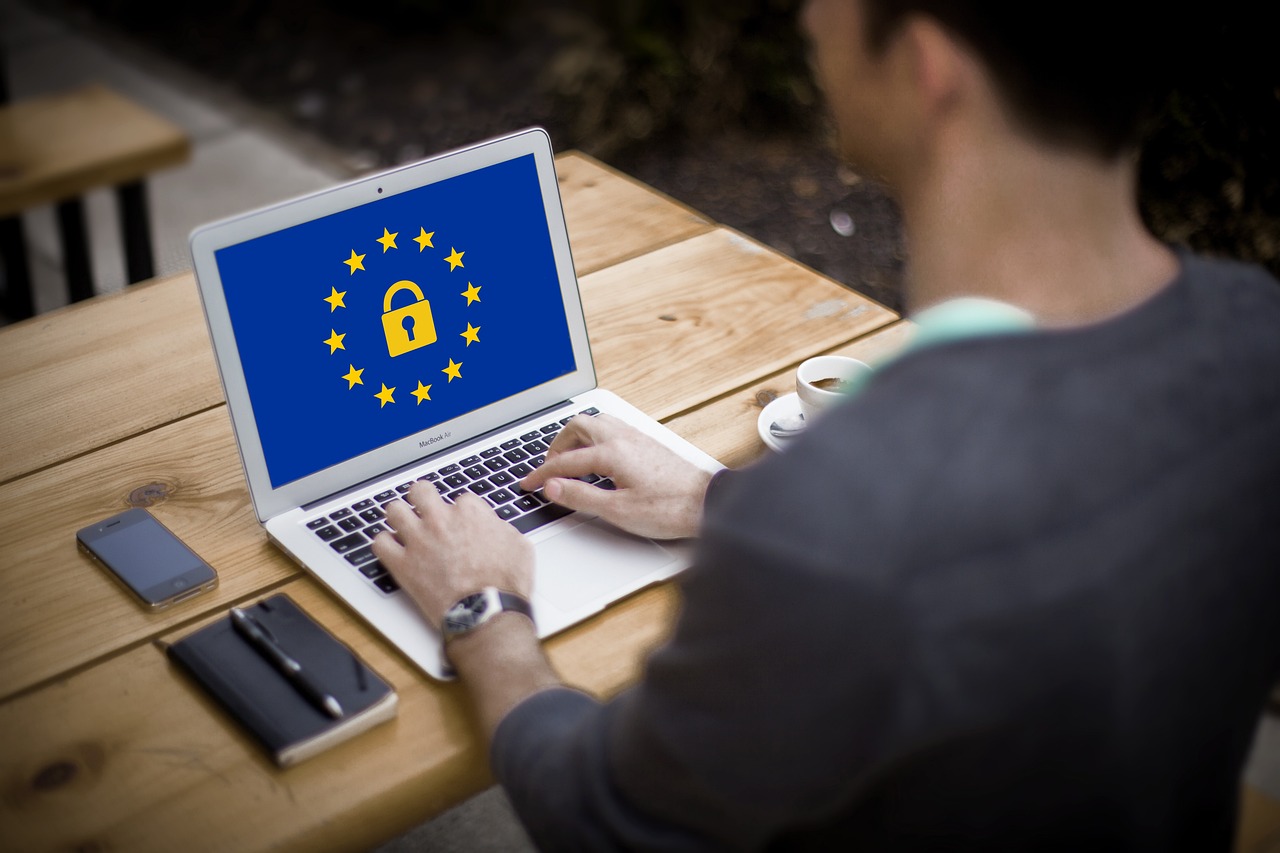
Large language models (LLMs) are becoming the bread and butter of modern NLP applications and have, in many ways, replaced a variety of more specialized tools such as named entity recognition models, question-answering models, and text classifiers. As such, it’s difficult to imagine an NLP product that doesn’t use an LLM in at least some fashion. While LLMs bring a host of benefits such as increased personalization and creative dialogue generation, it’s important to understand their pitfalls and how to address them when integrating these models into a software product that serves end users. As it turns out, monitoring is well-posed to address many of these challenges and is an essential part of the toolbox for any business working with LLMs.