How to Ensure Consistent Performance in Quant Trading Systems
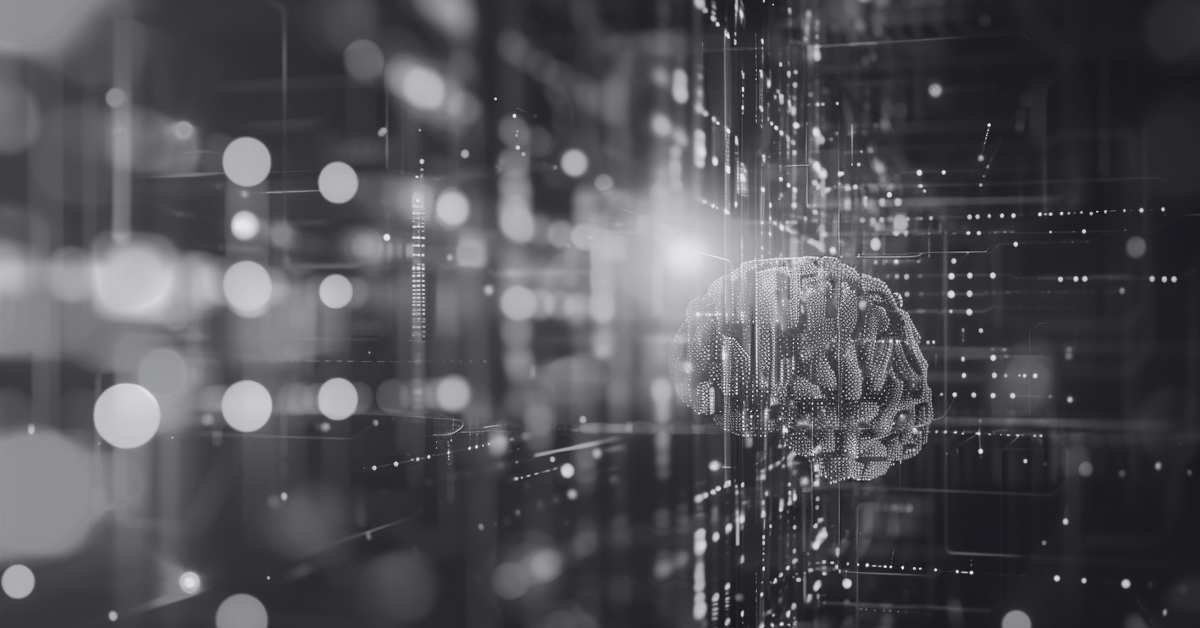
The Challenges of Algorithmic Trading
Automated quantitative trading systems are sophisticated, but they face a myriad of challenges that can disrupt their performance. From issues with data and models to internal operations, there’s a lot that can go wrong.
One of the biggest challenges is the reliance on third-party data sources. These sources can change without notice, causing disruptions that ripple through your entire system. Moreover, when you’re using different models and versions across various markets and asset classes, it becomes incredibly difficult to detect performance degradations (caused by a variety of things, e.g., data drift). The sheer volume and complexity of the data make identifying problems early like, “finding a needle in a haystack.”
Market behavior is inherently volatile, further complicating the process of distinguishing between real issues and noise. Unfortunately, many issues are only detected after they’ve already caused a decline in returns—by then, it’s too late. While most teams have some form of monitoring in place, it’s often standard, semi-manual, and reactive.
Efforts to deeply and automatically monitor these systems are hampered by a range of problems: big data challenges, organizational constraints, and most notably, the issue of false alarms, which can lead to alert fatigue within the team.